Statistical Toolbox: Hitting
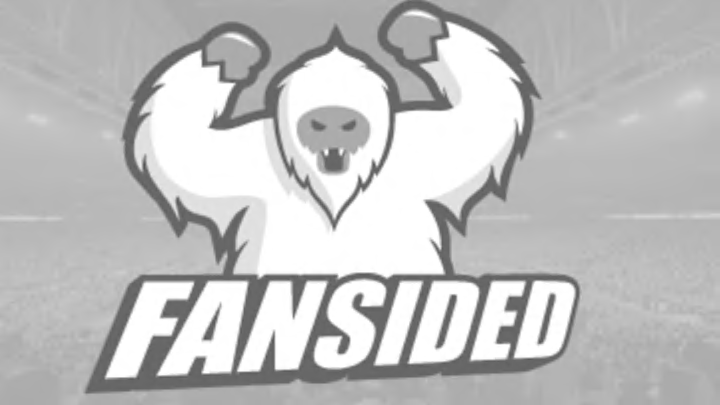
Credit: Chris Humphreys-USA TODAY Sports
I don’t know who you are. Do you spend your time contemplating the nature of the three true outcomes? Do you steal away at work to consider Michael Cuddyer’s true talent? Do you, reader, seek to understand the chasm between Jhoulys Cachin’s ERA and FIP, so that you might find a sustainable bridge? Do you even know what the hell FIP is? Do you know whether BABIP a noun or a verb? When I write about WAR, do I risk sounding enthusiastically bellicose?
I don’t know the answers to these questions because I don’t know who you are. You might be well versed in baseball statistics that I have never heard of, but you also might be unwittingly fettered to the averages, earned run as well as batting. And because I don’t know who you are, I’m going to dedicate a series of articles to breaking down and contextualizing what I find to be the most useful baseball statistics. They will also be the ones you’ll find in my writing throughout the season, as well as in the blogs of the other Rox Pilers. The posts are designed to be helpful references for those unfamiliar with these statistics; and for those already fluent in the metrics described herein, my hope is that you will find concise descriptions and examples from a slightly different perspective.
Statistics are explanatory and sometimes predictive tools that help us understand the relative value of individual players based on their skills. Think of the figurative toolbox like a literal one. Each tool has a different function to be used for a specific purpose—hammers hammer, screwdrivers screw. You also don’t need to know how to make a screwdriver in order to use it. I suck at math and will never create a baseball statistic. I’m also not equipped with a mathematical vocabulary. But that language isn’t necessary to use baseball statistics; that they require a mathematician to calculate shouldn’t be intimidating for the uninitiated. It is important, however, to know the function of a statistic so that it does not mislead. After all, you don’t want to use a screwdriver as a hammer. As Dave Cameron lucidly explains, the most important thing to know about every baseball statistic is that it is the answer to a question. It is important to know the question as well as the answer. Finally, because this is Rox Pile, I’m putting things in the context of the Rockies. This does mean more Michael Cuddyer pessimism.
Like a gifted sixth sense, statistics can be put to use in such a way that makes baseball more enjoyable. Today’s post is dedicated to hitting.
* * * *
Let’s start basic.
The Question: how frequently does a player get a hit (single, double, triple, or home run) in relation to how frequently he makes an out?
The Statistic: Batting Average (AVG), calculated by dividing hits by at bats
What it does and does not tell us: AVG gauges the valuable skill of getting on base by getting a hit. It is necessary to pay attention to batting average because it is foundational for a variety of other hitting statistics. But, as Andrew Martin notes, AVG does not account for a batter’s full profile. It is a metric that is equal parts forgiving and vindictive. First, it forgives a batter for sacrifice bunts and flies, even if a sacrifice to move a runner over was never the intent of the hitter. Sacrifices do not count as an “at bat.” Second, it maliciously does not give a batter credit for a walk, even though in many cases a walk is as good as a single. Walks and beanballs do not count as an “at bat” and thus do not change a hitter’s batting average. Additionally, AVG equalizes all hits. Home runs are just as valuable as singles in terms of AVG, but home runs are more valuable because they produce runs—the currency of victory—in every instance, whereas singles do not.
In Context: offensive production in Major League Baseball fluctuates, so where an AVG lands on a scale ranging from “stunning” to “unseemly” does not translate well over time. For example, in 2013 Michael Cuddyer won the National League batting title with a .331 AVG (Miguel Cabrera topped all of baseball with a .348 mark); Cuddyer and Cabrera were two of 24 qualified players to hit over .300, which is usually the boundary that separates the “good” from the “very good.” Conversely, offensive statistics were inflated in 1999, when Larry Walker won the batting title with a .379 AVG. That year, he was one of 55 qualified major leaguers to hit over .300. An AVG of .331 in 1999 would have been good for twelfth in all of baseball, which is obviously still quite good. Today, the major league average hovers around .260, so we can think of the context on the following scale (disclaimer: distinctions between levels are subjective, can easily overlap, and invite protest):
Metric | Stunning | Beautiful | Attractive | Quotidian | Drab | Unseemly |
AVG | .340 and above | .320-.339 | .300-.319 | .270-.299 | .241-.269 | under .240 |
* * * *
While batting average is a necessary statistic, not least because it is never going away, it does not tell the whole story. The purpose of our next metric is to help explain AVG.
The Question: how can we better interpret AVG as a skill based on things like speed, the opposition’s defense, and plain luck?
The Statistic: Batting Average on Balls in Play (BABIP), calculated by dividing hits on balls in play by at bats.
What it does and does not tell us: BABIP does not tell us anything without a corresponding look at AVG, nor does it indicate how well a player is or is not doing—but it can explain why a hitter is or is not doing well. By measuring only balls in play (thus excluding strikeouts and home runs, two of the “three true outcomes,” the other being walks), BABIP can account for the variety of oddities that we know happen all of the time: those bloop singles that probably should not have landed for hits, as well as those would-be doubles robbed by the likes of Nolan Arenado at the third base line. It is best used as a retroactive statistic to explain an anomalous AVG, but it can also have predictive value. If we take all balls in play for every game for an entire season, in general about 30 percent will fall for a hit, translating to a league-wide BABIP of .300. A player with a “normal” BABIP year generally lands between .290 and .310. This is an important baseline when looking at an individual player’s BABIP. If a player has a high BABIP of .350, we can usually expect it to regress toward .300, just like we can expect a low BABIP to move toward .300. In either case, a hitter’s will be affected AVG.
In Context: Let’s return to our 2013 National League batting champ, Michael Cuddyer, to serve as an example. Here is Michael Cuddyer’s AVG and BABIP by season (min. 300 at bats):
Year | AVG | BABIP |
2004 | .263 | .303 |
2005 | .263 | .309 |
2006 | .284 | .328 |
2007 | .276 | .315 |
2009 | .276 | .295 |
2010 | .271 | .298 |
2011 | .284 | .312 |
2012 | .260 | .287 |
2013 | .331 | .382 |
Credit: Christopher Hanewinckel-USA TODAY Sports
The anomaly is clear. In 2013, more of Cuddyer’s balls in play landed for hits than league average as well as his career average. We can say he was lucky, just like we can say that a hitter who finished with an unseemly AVG was unlucky if he BABIPed (it’s a noun and a verb!) .250.
BABIP comes with a caveat: the statistic without context is an incomplete story. We can only evoke “luck,” as Alex Skillin points out, after referencing another of Cuddyer’s statistics, his line drive rate (LD%). Of every type of batted ball—fly ball, ground ball, and line drive—line drives land for hits most often. If in 2013 Cuddyer dramatically increased his LD%, then we should conclude that he started doing something differently to produce more hits. But his LD% was about 20%, which is right in line with his career norm of about 19%. In contrast, Todd Helton finished his career with a BABIP of .330, partly due to a career LD% of 25%. Fast players also tend to have higher BABIPs because they can beat out a lot of ground balls for singles. So, Michael Cuddyer’s BABIP explains his batting title, but it also lets us predict with reasonable certainty that he is unlikely to repeat his 2013 performance.
* * * *
BABIP can help explain an aberrant AVG, but it does not compensate for what AVG cannot fundamentally explain.
The Question: how can we measure things that AVG doesn’t take into account, such as the value of extra base hits and walks?
The Statistic: in this instance, there are several metrics. On base percentage (OBP) and slugging percentage (SLG) usually accompany AVG to form a batter’s “triple slash.” OBP compensates for batting average’s failure to consider walks and is calculated by adding hits, walks, and times hit by pitch and dividing that number by plate appearances (not at bats); SLG makes up for AVG’s equalization of hits and is determined by dividing total bases (where a home run is four and a single is one) achieved by a hit and dividing that by at bats. Added together, the last two components of a triple slash form the literally named statistic on-base plus slugging (OPS). The most concise and easily understandable statistic, and the one I’ll focus on here, is OPS+. As far as I can tell, OPS+ is calculated by magicians.
What it does and does not tell us: OPS+ adjusts for variables by league and ballpark. It is more useful than OPS because of the easy to understand scale it inhabits. League average is always 100, so that an OPS+ of 110 is ten percent above league average, and a mark of 90 is ten percent below. It is a great statistic for comparing players. But because OPS is the equitable progeny of OBA and SLG, it also weighs the two statistics equally, and that is its limitation: the same sort of magicians that calculate OPS+ have determined that OBP is almost twice as important in terms of scoring runs than SLG.
In context: AVG is the foundation of both OBP, SLG, and it also plays a central role in OPS and OPS+. But OPS+ reminds us that in terms of scoring runs, AVG only tells part of the story. The batter with the highest average on a team is not necessarily the team’s best run producer. The following table shows the OPS and OPS+ for all 2013 Rockies with at least 400 plate appearances.
Player | OPS | OPS+ |
Carlos Gonzalez | .958 | 144 |
Troy Tulowitzki | .931 | 140 |
Michael Cuddyer | .919 | 137 |
Wilin Rosario | .801 | 105 |
Dexter Fowler | .776 | 102 |
Todd Helton | .738 | 90 |
Nolan Arenado | .706 | 82 |
DJ LaMahieu | .673 | 75 |
Credit: Chris Humphreys-USA TODAY Sports
The range of performance was pretty wide. Carlos Gonzalez’s OPS+ of 144 tells us that he was 44% better than league average. That’s good. Note that Michael Cuddyer’s .331 AVG was 29 points better than Gonzalez’s .302, and that his OBP was 22 points better, .389 against Gonzalez’s .367. However, according to OPS+ Gonzalez had the better offensive season, due to a better SLG. On the other end of the spectrum we have a frequent occupant and defiler of the two spot in the daily lineup, DJ LaMahieu, who produced 25% below league average. That’s bad.
Metric | Ambrosial | Perfumed | Fragrant | Odoriferous | Malodorous | Putrid |
OBP | above .400 | .360-.399 | .330-.359 | .310-.329 | .290-.309 | under .290 |
SLG | above .600 | .500-.599 | .450-.499 | .400-.449 | .350-.399 | under .350 |
OPS | above 1.000 | .900-.999 | .800-.899 | .750-.799 | .700-.49 | under .700 |
OPS+ | above 150 | 130-149 | 110-129 | 90-109 | 75-89 | under 75 |
* * * *
Because OPS and OPS+ give equal weight to OBP and SLG, it is also an incomplete statistic.
The Question: What is a player’s total offensive production—just one number, please?
The Statistic: Weighted on Base Average (wOBA), which honors the inequity of triples and singles according to their run value and is computed by sorcery—real dark stuff.
What it does and does not tell us: developed by Tom Tango, wOBA ostensibly tells us everything as far as production from the plate. The statistic is designed to consider every aspect of hitting according to a given hit’s ability to produce a run; it accounts for unintentional walks, too. It is the most commonly used comprehensive statistic. Whereas AVG and OBP equalize all hits, SLG differentiates them in a misleading manner (it is incorrect to say that because a double is two total bases and a single is one, it’s twice as important, but that’s what SLG does), and OPS assumes that one OBP point is the same as one SLG point, wOBA weighs plate appearance outcomes, hits as well as the ability to earn a walk, proportionally to a given outcomes ability to create a run.
In Context: in a previous post, I noted that wOBA is scaled similarly to OBP, so in addition to measuring offensive production completely, it is easy to understand. Rockies fans will not be surprised to find out that the team had two players break the lofty .400 wOBA barrier in 2013: Carlos Gonzalez’s .408 and Troy Tulowitzki’s .400 were the two best marks in 2013. In Rockies history, Larry Walker has the highest wOBA with a transcendent .440 wOBA with the club. Finally, another statistic, weighted runs created plus (wRC+) is derived from wOBA. It’s based on the same principle about proportional ability for a certain type of hit to create a run, but it is scaled the exact same as OPS+. So DJ LaMahieu’s wRC+ of 70 in 2013 was 30% below league average. To the table, all previous disclaimers apply:
Metric | Transcendent | Sublime | Worldly | Common | Numbed | Anaesthetized |
wOBA | above .400 | .360-.399 | .330-.359 | .310-.329 | .290-.309 | under .290 |
wRC+ | above 150 | 130-149 | 110-129 | 90-109 | 75-89 | under 75 |
* * * *
In sum, this overview should demonstrate that no statistic is a panacea, not even wOBA, because they all interact in some fashion. Every statistic has its limitation, but as long as the limits are recognized, they can remain useful in answering a variety of questions. That also means that no statistic should be viewed in isolation. Being mindful of a given metric’s purpose and deficiencies gets us closer to a total baseball experience.
Metric | Outstanding | Exceptional | Admirable | Satisfactory | Deficient | Terrible |
AVG | above .340 | .320-.339 | .300-.319 | .275-.299 | .241-.274 | under .240 |
OBP | above .400 | .360-.399 | .330-.359 | .310-.329 | .290-.309 | under .290 |
SLG | above .600 | .500-.599 | .450-.499 | .400-.449 | .350-.399 | under .350 |
OPS | above 1.000 | .900-.999 | .800-.899 | .750-.799 | .700-.49 | under .700 |
OPS+ | above 150 | 130-149 | 110-129 | 90-109 | 75-89 | under 75 |
wOBA | above .400 | .360-.399 | .330-.359 | .310-.329 | .290-.309 | under .290 |
wRC+ | above 150 | 130-149 | 110-129 | 90-109 | 75-89 | under 75 |
I invite quibbles, suggestions, questions, and corrections in the comments or on twitter. Next week, I’m going to cover pitching statistics, which means that we’ll take a week off from disparaging Michael Cuddyer. Although I’m sure his fastball is terrible.
Resources:
The following resources are excellent and highly recommended: